October 6, 2023
The story goes that an angry father confronted Target employees after his daughter was mailed coupons for maternity products unnecessarily, only to find out later that she was pregnant. A triumph of big data combined with statistical learning, and a creepy portent of the future, right? That’s how the story went at least.
Or, perhaps Target realized the cost of false positives (sending coupons to the wrong person) is the negligible commercial mail rate, whereas a false negative means potentially missing out on sales of a huge volume of maternity and childcare products1. This surveillance incentive can lead to seemingly miraculous results from mediocre inferences.2 The “big data” inference line made great media copy, though, and so the story became embedded in the early 2010s tech zeitgeist—Big data are powerful. Private enterprise can give you what you didn’t even realize you needed based solely on computational inferences from scattered data about you and your habits.
The decade of experience since has proven just how wrong this was.
Ad targeting is mediocre—the famous 2009 Netflix prize was to reduce recommendation error 10% from very bad to slightly less bad. Netflix soon removed its star rating system for movies: the data collected was not particularly useful. Its catalog has shrunk by 80% since, suggesting that guiding users through the cinematic universe based on targeted recommendations is not a good business model3. Moreover, detailed analyses of the Cambridge Analytica scandal showed that the resulting inferences based on Facebook likes did not improve on previous voter targeting methods that did not use social media data.
More prosaically, if you visit an online jewelry store to buy an engagement ring, an archetypical “one time purchase,” you will be barraged with ads for additional engagement rings for months. This is not a sign of valuable, sophisticated inference.
Indeed, the only place ad targeting seemed to work consistently well was Amazon’s book recommendation engine back when it was still a bookstore. As the volume of products offered expanded, the utility of recommendations plummeted. In a small, closed universe where the main way people interacted with a product category was through your service, good inference was maybe possible. In an open world, even basic demographics are hard to infer: you can see what Google predicts about you here. Despite using broad age, ethnic, and gender categories, it is still wrong remarkably often.
Years of techy hype around “big data” has led to closed ecosystems predicated on proprietary data being useful. Public evaluation of models is impossible when datasets are closed and hidden. Lived experience (and the few public competition results we have) suggests that they are not performing well at all.
Why is this? Humans are variable and capricious—and the signal from most data is low. Data are collected in diverse, non-standardized ways, and merged together with a wing and a prayer. Or as one data scientist put it5:
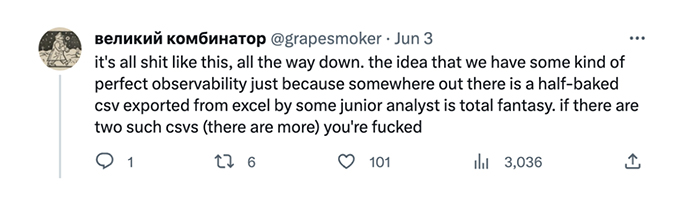
Big data is not a panacea. Merging disparate datasets all too frequently becomes garbage in, garbage out. Classic pathologies include:
• Inconsistent naming of objects: Think of street names and addresses. What’s the format? What’s the abbreviation you use? Do you remember what’s technically a street vs a road vs an avenue? What do you call highways anyhow? [I-]95, [Route-]128, Blue Star Memorial? Is it an expressway or a highway anyhow?
• Inconsistent measurement of objects: What are the units? How do you record velocity: is it a speed and direction? A line/vector? Words versus numbers? What is the precision?
Interactions between objects: Are they recorded or inferred based on colocation and simultaneity? Are they in the same system? Do they have the same information about each participating object?
• Inconsistent ontologies: Information is arranged hierarchically. Two entities can agree at one level but not another. At EQRx, we’d work with all manner of records about doctors and hospitals that disagreed on which clinics a doctor was primarily affiliated with as well as which hospital they belonged to, not to mention the health systems that owned them and the business addresses for each entity.
• Incompleteness: We all too often assume that missing records are few and distributed identically to those we possess based on scanty evidence. Even when the missingness is known, it is difficult to determine whether absence of those data affects sampling and ability to draw inferences from data4.
Every dataset has a set of conventions tied to the reason it was collected in the first place. Merging and creating “big datasets” implicitly breaks these conventions by tying multiple ones to one another. It is unlikely that they will match with high fidelity. This phenomenon is a direct result of lack of standardization and digitization. Attempting to draw inferences from merging datasets like this unsurprisingly does not work very well in general.
That’s just tabular data. So much information is locked up in pdfs that cannot be consistently converted to digital text (OCR) and read. While text recognition algorithms and Large Language Models (LLMs) have made some improvements here, we see only the positive examples and not the legions of failures. Formats are inconsistent. Data live in poorly annotated tables that stretch across pages in varying styles, not to mention their placement in text.
The world is full of information we cannot reach, record, or infer. We try to superimpose modernity on a complex, non-digital world, where every metaphorical screw uses a different thread size, and the numbers corresponding to their diameters are on completely different scales. The only solution is to control the process and build reproducible standards.
And so there has been a quiet pivot to closed data universes and a panopticon of ceaseless tracking. If you cannot publicly justify your big data hype, make sure no one can use your data to show how poorly your algorithms work, or worse, do better at inference than you. If statistical inference and Machine Learning fail to predict meaningful characteristics, it’s better to collect as much physical information as possible on your customers so you have as many channels as possible on which to try to contact them.
Today we live in a world with endless cookies and copying of search history with no privacy in internet usage. A liquid market in private data brokers (e.g., Acxiom / Liveramp) will sell your address, phone number, and any plausible records about you to anyone interested. Unsurprisingly, trust is broken. Strong hype about big data (in the press and in investments), little public understanding about its limitations, and a barrage of poorly targeted advertisements are a toxic combination.
The possibility of ultra-rare re-identification, such as the miniscule probability someone could be identified in the Netflix competition dataset by matching Netflix reviews to IMDB ones is treated as a reason to shut everything down, not as a starting point for debate. Novel consumer technological offerings and services come under a thicket of regulatory scrutiny even when they provide great benefits to its users. Meaningful public data collection exercises, such as the Census come under threat and are rendered useless by overly strong anonymization procedures that bury signal among fake data for downstream users.
Big data is not a panacea; it’s often surprisingly useless. If only this were the story the public was sold back in 2012, society would be better poised to make meaningful decisions around new digital technolog —and organizations better able to understand the importance of digitization before jumping headlong into data science and machine learning.
—————
1An excellent mathematical and sociology breakdown of this story can be found here.
2In technical terms, maximizing recall without regard for precision.
3I have drawn considerable inspiration from this essay by Ben Recht.
4A classic example is open (transactional) claims data. Large sections of the US healthcare system are transacted through private networks that are not present in these datasets, including the VA, and integrated payer-provider systems like Kaiser. Similarly, doctors seeing patients in clinical trials appear rarely in these data despite their importance. Drawing appropriate inferences is hard, as I discuss here.
5I highly recommend reading the entire (somewhat profane) thread to better understand the life of a data scientist.
To subscribe to Engineering Biology by Jacob Oppenheim, and receive newly published articles via email, please enter your email address below.